Be intentional about including the following tactics in your new year marketing strategy As 2025…
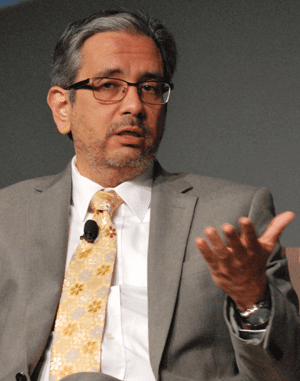
Data analytics still need the human touch for metalworking equipment marketing
By Mike Bacidore, chief editor, Control Design, Nov 17, 2015
Data is everywhere, and many manufacturers have been collecting it for decades. But what are they doing with it? Can you collect enough data to create an analysis algorithm that replaces the need for human intervention?
“We don’t need big data,” asserted Francisco Castillo (shown), chief information officer, Maynilad Water Services. “Most of our data is field data, and it’s quite repetitive, so we can compress it. For us, to do a good analysis of the information, we need someone who is knowledgeable of the process itself. That is more of a challenge.”
Castillo was one of a group of panelists who spoke about data analytics during the Automation Perspectives media event in the run-up to Automation Fair this week in Chicago.
Different vertical industries have different views of data, but they all seem to agree on the need to understand the process – and its potential impact on developing their own Connected Enterprise.
“Data is data,” said Wayne Roller, engineering director at 3M. “But you need a ton of data to run an analysis, unless you’re very intimate with how that process works. Having intimate process knowledge with a small amount of data, you can move the world. The key is getting it down to the user level with analysis that can be run quickly.”
That ability to combine data with process knowledge is a winning formula for analytics.
“Data does not replace the cognitive capability of a human being,” explained Matthias Altendorf, chief executive officer, Endress+Hauser. “But data can help you recognize patterns. The data of the device and the data of the environment can help a human being come to a decision faster.”
More than one way to get to the data
At Fanuc America, the robot manufacturer found itself catapulted into data acquisition and analytics by its best customer.
“I’d characterize our journey as a slingshot being launched,” said Rick Schneider, Fanuc president and CEO. “We had a certain component that would fail in a machine that was in the middle of a production line. It would take four to six hours to take down the production line and replace it.”
With this type of failure costing its customer $2.5 million in downtime per incident, Fanuc acted fast to determine how to get at the data and use analytics to slash that downtime cost.
“We put together a test site for the highest-risk machines,” explained Schneider. “We started pulling the data out, and we had our engineers go through it to look for symptoms that occurred before the failure.”
Each machine contained a black-box recorder. Fanuc started pulling data out every 90 seconds, and it quickly found it could predict and prevent failures with that information, saving its customer an estimated $30 million in avoided downtime.
“All of that has happened in less than a year,” said Schneider. “For us, it’s been a very fast ramp-up, and the investment cost has been very low.”
At Maynilad, the journey was much slower, but the challenges were different.
“One of the things that is a reality for us is the convergence of IT and OT,” explained Castillo, who oversees both departments, with OT under the management of IT. “We now have hydraulic modeling—a core system in the water industry—to simulate how an expansion in one area will affect water delivery. In one of our plants, we’re able to capture operational data and notify heads before a failure occurs. Plus, we’ve been able to upgrade our leak management system.”
Because the water industry is so highly regulated, the only way to increase profits is to reduce costs. Maynilad uses data from both IT and OT. It uses field data, but also incorporates asset information and billing information for a complete picture.
“Most of our assets are underground,” said Castillo. “We want to deliver pressure, but not too much because that can increase leaks. We’re upgrading and installing sensors to detect more than the traditional signals, so we can now measure temperature, vibration and current, and be able to do condition-based maintenance.”
3M has been collecting data for many years, but it still comes back to human knowledge of the process.
“As instruments got cheap enough for us to add data collection, we started charting information and getting it in front of our operators,” said Roller. “But, without some model-based understanding of the process, you’re still chasing ghosts. We’re now moving toward a more model-based approach to understand why the data doesn’t look like it should. We’re still trying to do that in real time on the production floor. Then we’re taking that data up to our own servers and running big data analysis.”
At Endress+Hauser, the journey started about 20 years ago because the company has so many manufacturing sites around the world.
“For 15 years, we’ve collected all of the data for manufacturing,” explained Altendorf. “Now we have more than 30 million data collection devices.”
Where the data lives
While many tend to think of analytics occurring in the cloud, more manufacturers prefer to do computing and analysis at the edge. Each strategy provides its own challenges.
“Analytics is about contextualizing data in terms of actual business problem,” explained Blake Moret, senior vice president, control products & solutions, Rockwell Automation.
“In the past, we’d do edge computing, and we’d work with Rockwell on cloud computing, and in some cases we’d pull that data out,” said Schneider. “The plant didn’t want us to know what was going on in that cell. We would plug in a box to collect data, and we’d come back and find the box had been unplugged. The plant’s IT department didn’t want us pulling that data out.”
Fanuc then worked with Cisco to put the data on a network that it could access, but then it had to go plant by plant and country by country, a very slow process.
Data connectivity and analytics have changed Fanuc’s go-to-business model.
“After we’ve sold that robot to the customer, we have very little insight as to how that robot is being used,” explained Schneider.
In the past, it only found out something when the robot was down. “We want to be at the customer’s plant within six hours,” he said. “Now that we’re getting feedback from that robot every 90 seconds, we’re able to get a lot of data.”
Fanuc is able to tell the customer how to reduce energy consumption or how to run a robot in a manner that will cause less damage to it. And it’s improved customer satisfaction and generated more profitable post-sales service opportunities.
“As long as the data is there, you might as well store it,” offered Castillo. “Once you look at the process, you might find you need more data, so it’s beneficial to have it then. Data governance, or data ownership, is a big challenge for us. Who owns the data? How do you keep it clean? Who owns not only the inputs, but the outputs? We came up with a data governance model. We put in as much data as possible to prove something good can come out. That’s a work in progress.”